Parameter identification for a local field potential driven model of the parkinsonian subthalamic nucleus spike activity
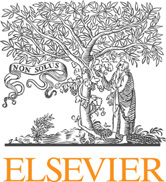
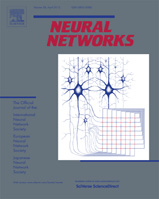
Contents lists available at
journal homepage:
Parameter identification for a local field potential driven model of theParkinsonian subthalamic nucleus spike activity
Kostis P. Michmizos Damianos Sakas , Konstantina S. Nikita
a Massachusetts Institute of Technology, Cambridge, MA, USAb Evangelismos Hospital, Department of Neurosurgery, National and Kapodistrian University of Athens, Athens, Greecec National Technical University of Athens, Athens, Greece
Article history:
Several models, with various degrees of complexity have been proposed to model the neuronal activity
Received 18 October 2011
from different parts of the human brain. We have shown before that various modeling approaches,
Received in revised form 9 October 2012
including a Hammerstein–Wiener (H–W) model, can be used to predict the spike trains from a deep
Accepted 10 October 2012
nucleus, the subthalamic nucleus, using the underlying local field potentials. In this article, we present,in depth, the various choices one has to make, and the limitations that they introduce, during the H–W
model parameter identification process. From a segment of the recorded data, which contains information
Subthalamic nucleus modeling
about the spike times of a single neuron, we identify and extract the model parameters. We then use those
Nonlinear modelMicroelectrode recordings
parameters to numerically simulate the spike timing, the rhythm and the inter-spike intervals for the rest
Parkinson's disease
of the recording. To assess how well the model fits to the measured data we combine measures of spiketrain synchrony, namely the Victor–Purpura distance and the Gaussian similarity measure, with time-scale independent train distances. We show that a wise combination of metrics results in models thatpredict the spikes with temporal accuracy ranging, on average, from 53% to more than 80%, depending onthe number of the neurons' spikes recorded. The model's prediction is adequate for estimating accuratelythe spike rhythm. Quantitative results establish the model's validity as a simple yet biologically plausiblemodel of the spike activity recorded from a deep nucleus inside the human brain.
2012 Elsevier Ltd. All rights reserved.
brain are carried by volleys of spikes arriving almost simultane-ously to multiple dendrites of a neuron
Understanding information flow inside the brain pathways and
These volleys give rise to low frequency (below 150 Hz)
how this is altered in the case of a disease is a basic endeavor
postsynaptic activities in neurons, and are believed to comprise a
in several different fields. Understanding information flow inside
significant part of a particular class of electrophysiological signals,
the central nervous system building block, the neuron, is one of
namely the local field potential (LFP)
the fundamental problems of neurophysiology; the development
of neuromodulation surgical procedures, such as deep brain stim-
A question arises about whether one can use an LFP-driven
ulation (DBS) that alleviates symptoms of motor related diseases,
model to accurately simulate the brain activity in terms of spike
requires a thorough understanding of both how the neuron nor-
timing and rhythm. That predictive relation between LFPs and
mally functions and how this may best be introduced to a biophys-
spikes, per se, is starting to attract great attention. Spike predic-
ically accurate brain model. The work presented here takes part in
tion has been firstly examined in the primary visual cortical area
an attempt to bind together two of the most important hypotheses
(V1) using extracellular microelectrode recordings (MERs) in anes-
on neurons' language; the ‘‘ruling school of thought'' which bases
thetized monkeys
all descriptions of the brain on the average spike rates, treated
A recent study in the primary somatosensory cortical area
as functions of time, and the, now growing attention, aggregate
(S1) of rats using a combination of intracellular and extracellu-
dendritic activity according to which the meaningful signals in the
lar MERs reveals an analogous interconnection between LFPs andspikes In addition to proving that
spikes can be predicted by the area's LFP, the opposite is also sug-
Correspondence to: Massachusetts Institute of Technology, Room 3-147, 77
gested: some of the local properties of the LFPs are predicted by
Massachusetts Avenue, Cambridge MA 02139-4307, USA. Tel.: +1 617 253 8114, +1857 756 4599; Mob: +1 857 756 4599, +1 857 756 4599.
the spiking activity of a few or even a single neuron in the V1
E-mail address: (K.P. Michmizos).
of anesthetized monkeys
1 Tel.: +30 210 772 2285; fax: +30 210 772 2320.
Using MERs from the subthalamic nucleus (STN) of Parkinson's
0893-6080/$ – see front matter 2012 Elsevier Ltd. All rights reserved.
K.P. Michmizos et al. / Neural Networks 36 (2012) 146–156
disease (PD) patients, we have shown that the spike activity of a
generation decision. This cascade model is referred to as the
deep brain nucleus can also be predicted from the LFPs. Various
Wiener model.
nonlinear models are used as spike predictors, including a Ham-
As any current flowing through a resistor results in a voltage
merstein–Wiener (H–W) model
drop, the input signal (LFP and noise) is attenuated as it propagates
through the dendrites towards the soma. For a dendrite to be mod-
a modified LFP-driven Izhikevich model
eled as a (linear) resistor, strict assumptions such as constant di-
a simple bi-exponential model
ameter and homogenous distribution of ion channels are required.
and a Volterra model
Yet dendrites are complicated structures and the observed volt-
age attenuation, due to known and unknown dendritic processes,
The development of brain models, either using biophysically
is far from being regarded as linear. The nonlinear voltage drop
accurate Hodgkin–Huxley-type neuron models or computationally
was introduced to the model with a second nonlinear static pro-
efficient integrate-and-fire neuron models, usually remains on
cess before the Wiener system. The overall system was a Hammer-
a large-scale level. Consequently, in most of the experimentally
stein–Wiener model to be mathematically presented in Section
verified models of the basal ganglia (BG) (e.g.,
Processing and analysis of the MERs to be used in simulations is
presented in Section
the STN, which is of interest in this study, is a peripheral structurewith its neurons modeled very simplistically. In fact, only few
2.1. Data acquisition
computational models of the STN neuron itself currently existand are mainly based on anatomical data
Data were taken from recordings partially presented elsewhere
Although the STN is believed to possess a pivotal role in BG
Extracellular MERs, recorded from three
unmedicated, awake PD patients, during DBS implantation, were
included in the present study. Recordings were acquired during pe-
its underlying networking
riods in which the patient lay down immobile in the operational ta-
mechanisms are still partly unknown
ble, prior to DBS permanent lead implantation. MERs began above
The extraordinary rich and varied range of firing patterns
the estimated upper border of the STN and allowed for a complete
that the STN cells are able to produce have
sampling of the neuronal activity of the STN. They were obtained at
not been faithfully modeled yet.
0.5 mm (or fewer) steps through the STN to the substantia nigra us-
Given the importance of the STN and the possible use of the
ing the LeadpointTM Software, Medtronics Functional Diagnostics
LFP-driven models as test beds for current hypotheses on the effect
S/A. For each electrode depth, 10 s recordings, digitized at 24 kHz,
of DBS on the cellular level we now
were simultaneously acquired from 5 electrodes positioned in a
seek to formalize the evidential modeling paradigm to a concise
cross (Ben-Gun) formation. The distance between any peripheral
identification scheme for the selection of the optimal parameters
electrode and the central one was 2 mm. After visual inspection for
for MER-specific H–W models. Herein we propose a prediction-
excluding MERs with artifacts or without the typical discharge pat-
error minimization method to estimate a set of H–W models
tern of an STN neuron
that predict the recorded spikes from the LFPs acquired from the
the signals were low-pass filtered, using an FIR least squares
same or adjacent electrodes. To select the best possible model, we
filter with a cut off frequency of 6 kHz, order of 1300 and peak to
propose a two-step validation procedure: an exploratory analysis
peak (p–p) rippling equal to 8 × 10−9 db. The filtered signal was
based on time-independent metrics is followed by a confirmatory
then downsampled to 12 kHz for computational convenience.
analysis. The latter uses single-valued metrics derived from time-
A filter bank with 2 filters was applied to each MER to acquire
dependent measures that estimate the degree of synchrony
the LFP and spike signals. To acquire the LFP signal, VL, an FIR
between spike trains. By looking at the distribution of the spike
equiripple LP filter with a pass-band of [0 170] Hz and a stop-
rhythm residuals, we were able to show that the proposed
band of [0.22 12] kHz was used. To acquire the spike signal, Vs, an
identification procedure results in optimal models.
FIR equiripple band-pass (BP) filter with stop-bands of [0 430] Hzand [1.55 6] kHz and a pass-band of [0.48 1.5] kHz was used. Bothfilters had an order of 2351 samples, a p–p rippling of less than
2. Materials and methods
2 × 10−7 db and a constant over all frequencies phase delay of0.615 rad. Spikes were identified from the Vs, using amplitude
Although being regarded as a ‘‘black-box'' model, the H–W
thresholding The absolute value of the threshold
model has some physiological analogies between its structures
was typically chosen equal to 3SD of the distribution of all the sam-
and central parts of a neuron. The biological foundation of the
ples in the noisy spike signal, excluding the 1 ms spike segments.
model is discussed in detail in Briefly,
Qualitatively, this means that a V
there are evidences that LFPs recorded inside the STN reflect
s peak was considered as a spike
if its amplitude was almost 1.5 the noise level of the recorded sig-
synchronized aggregate activity of local STN neurons. In other
nal (95% confidence interval). This value assured that the peak sur-
words, a significant component of the recorded LFP arrives from
passed the noise level enough for an observer to be able to visually
a narrow STN area and hence can be used as a predictor for the
verify the existence of a spike, but did not eliminate the possibil-
STN spikes. Our hypothesis is further supported by experimental
ity of ‘‘hiding'' some spikes inside the noise signal, especially for
findings in cortical neurons according to which a large part of
multi-neuron MERs. As a final processing step, V
the LFP signal can be reconstructed from just a single neuron
s was binarized by
placing a 1 at the timing of each detected spike, defined by its max-
spike recording For modeling the LFP signal
processes that take place inside the cell's body and particularlyin the axon hillock, a linear time invariant (LTI) system was used.
Conversely, generation of spikes is a strongly non-linear process
2.2. The H–W model as a neuron model
since an abrupt change in the membrane potential precedes theopening or closing of ion channels. Thus, the LTI system was
The mathematical presentation of a lumped parameters H–W
followed by a nonlinear static module used to model the spike
model follows. If k = n · 1t, n ∈ N and 1t is the sampling period,
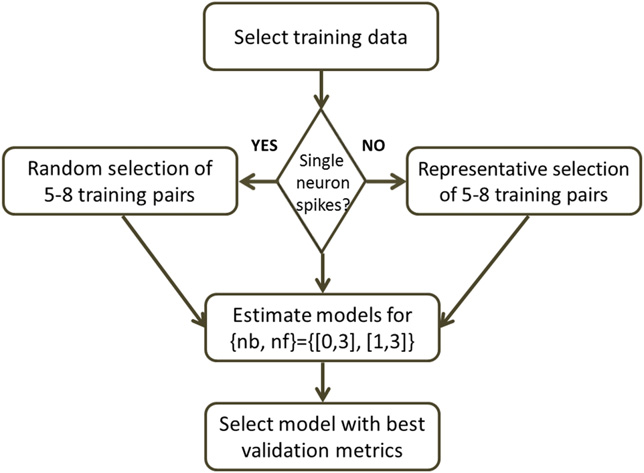
K.P. Michmizos et al. / Neural Networks 36 (2012) 146–156
such a H–W model is represented in Z space by
v(k) = f (u(k))
z−1 + · · · + γ z−nb
v(k) = v(k)
a′ + β′z−1 + · · · + γ ′z−nf
y(k) = h(w(k))
where u ∈
is the input (the LFP and the background noise)
to the entry module of the model. Input u is passed through thenonlinear mapping f (u) to give the input v ∈
dynamic block (Eq. In Eq. parameters α, β, . . , γ , and
α′, β′, . . , γ ′ are the polynomial coefficients for the numerator, B,and the denominator, F , of the linear dynamic block, respectively,nb and nf being the order of B and F , respectively. In Eq. w ∈
R is the output of the linear block which is passed through thenonlinear mapping h(w) to give the output y ∈ p
R of the model.
2.3. Identification of the H–W model
Fig. 1. Schematic representation of the identification steps for a MER-specific
model.
Recording-specific models were identified using an iterative
w, included a percentage, p, of the samples before the time of the
prediction-error minimization method. The nonlinear functions
peak and 1 − p of the samples after it. Selection of p and w will
f and h and the LTI were adjusted to the data to minimize the
be discussed in Section Each of the training pairs was visu-
simulation error signal, expressed as
ally inspected to avoid the presence of a second spike in the train-
ing pair. In single-neuron MERs, selection of the LFP-spike training
(y (u, t) − ϕ(t))2dt
pairs was random and a model of a single neuron was estimated. In
the multi neuron category, we applied K -means clustering via prin-
where ϕ was a scalar function representing the binary spike signal
cipal component analysis to relate each spike to a specific neuron.
over time, t. The integral was discretized using the Gaussian
Then the pairs were chosen in analogy to the percentage of each
quadrature formula and Eq. was formulated
neuron's spikes present in the recording. For example, for a MER in
as a least squares problem:
which 2 different neurons were firing with almost equal rhythm,
the training data would be composed of half pairs that belong to
F (u) = min f (u) =
the first neuron followed by the other half pairs belonging to the
i) − ϕ(ti))2
second neuron. In other words, in multiple-neuron MERs, a modelwas estimated, as if there was a single neuron firing all the spikes
where y and ϕ included the weights of the quadrature scheme and
that we recorded from the 2 -or more- neurons. The steps for pa-
the interpolating function, x, is a polynomial of degree 2m − 1 or
rameter estimation are summarized in
less. The residual ∥F (u)∥ was likely to be small at the optimum,
Typically, model orders and delays were selected by trial and
if we had set realistically achievable target trajectories. Follow-
error until a model that produced a satisfactory fit to the data was
ing initialization, the optimum model parameters (Eqs.
found. A satisfactory model predicted at least 50% of the recorded
were estimated using a prediction-error minimization method as
spikes in a 1 s segment of the validation data, without fabricating
follows. If we denote the m-by-n Jacobian matrix of F (u) as J(u),
more than 10% of non-existent spikes. Piecewise linear functions,
the gradient vector of f (u) as G(u), the Hessian matrix of f (u) as
known to provide the means to represent any nonlinear function,
H(u), and the Hessian matrix of each Fi(u) as Hi(u), we have
were used to approximate both f and h. The number of linear
G(u) = 2J(u)T F (u)
segments was kept equal to 10. Thus, only nb and nf needed to beestimated a priori. The ranges of their values were [0, 3] and [1, 3],
H(u) = 2J(u)T J(u) + 2Q (u),
respectively. Consequently, 12 H–W models were identified, using
where Q (u) = m F
the same LFP-spike training pairs.
i(u)Hi(u). The matrix Q (u) has the prop-
erty that when the residual ∥F (u)∥ tends to zero as u
Two iterative searching algorithms were used to estimate the
k approaches
the solution, then Q (u) also tends to zero hold Thus
model parameters (Eqs. under the assumption that the
when ∥F (u)∥ was small at the solution, a Gauss–Newton direction
parameters remained constant over the time of the recording. Pa-
could be used for the optimization procedure
rameters were initially set to 0 and a maximum of 50 iterations
Hence, a search direction, d
of the adaptive Gauss–Newton prediction-error minimization
k, was obtained at each iteration,
k, that was a solution of the linear least-squares problem:
method with no robustifi-cation, were used to train the model (Eqs. The iterative
dk = min ∥J(uk) − F (uk)∥2.
search was terminated when the expected improvement of the pa-
rameter values was less than 0.01%. When the second-order termQ (u) is significant, the Gauss–Newton method does not result into
2.4. Parameter estimation process
a valuable model. This was the case when the model was overpa-rameterized or the data was not informative enough and the as-
Each model was trained in a predefined MER segment with
sumption of invertibility for input and output nonlinear functions
a process that depended on the number of different neurons
did not hold (see This situation was identified when
recorded. The first 0.5 s for each [VL, VS] pair was detained for
either dk went below e−15 or when the condition number of J(uk)
model training and the remaining 9.5 s of the data were used
was below e−10 and the Levenberg–Marquardt method
for validation. For each spike present in the first 0.5 s of the VS,
was chosen as the iterative searching al-
a window was applied to both VL and VS. The window, of length
gorithm. The condition number of a matrix is the ratio of the largest
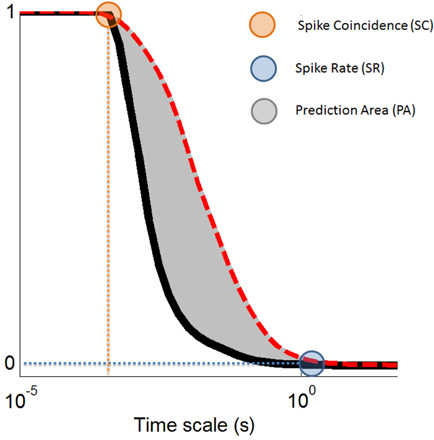
K.P. Michmizos et al. / Neural Networks 36 (2012) 146–156
singular value to the smallest. The estimated model parameters foreach LFP-spike pair were becoming the initial values for the nextrepetition of the identification procedure (model refinement).
2.5. Validation metrics of spike train synchrony
To quantify the performance of our proposed models, we
sought to characterize the agreement between y and ϕ by meansof the timing of individual spikes. Even in single-neuron MERs,recorded STN neurons exhibited a highly variable firing patternranging from bursting to pacemaker mode whereas spikes fromirregularly firing neurons were also present. Since no particularcoding pattern could be assumed, general measures suited foran exploratory analysis were followed by a set of redundantsingle-valued metrics for a confirmatory analysis. Specifically,we combined parameter-free and time-independent approacheswith the most widely used time-scale dependent measures. These
Fig. 2. Schematic representation of the single-valued metrics derived from the V–P
measures were complementary in nature and spanned throughout
distance (normalized spike distance metric). The estimated V–P distance betweenpredicted and recorded spikes (thick line) is compared to that between a random
the entire spectrum of measuring spike synchrony, from spike
prediction with the same spike rate (dotted line). For each prediction, the temporal
coincidence detectors to rate comparison.
resolution in which we found the Spike Coincidence (SC) and Spike Rate (SR) points
General parameter-free and time-independent metrics inclu-
and the Prediction Area (PA) determine the quality of the prediction. The V–P
ded (a) the plot of the empirical cumulative distribution function
distance for random prediction was averaged over 30 V–P distances estimated fromrandom spike trains.
(CDF) of ϕ against the CDF of y (b) a sorted interspike interval (ISI) plot for ϕ and y
interval for which two spikes can be considered to be related by
and (c) the rhythm
jitter; otherwise they better are deleted or inserted. The similarity
estimation in fixed size bins. For maximizing the CDF's temporal
measure uses a smoothing process with a controllable temporal
resolution, we defined the coincidence window as the minimum
size, τ = 1 sample. If the model accurately describes the observed
Both y and ϕ are convolved with a Gaussian function of a
spiking data, then the empirical and model CDFs should roughly
standard deviation ˆ
σ , which essentially determines the temporal
coincide, and the plot follows a 45° line. If the model fails to ac-
resolution used in the comparison. A large value means low
count for some aspect of the spiking behavior, then that lack of fit
temporal resolution and a small value means high temporal
is reflected in the plot as a significant deviation from the 45° line.
resolution. In this study, ˆ
σ varied from 0.5 to 6.7 ms. After
For the ISI method, the predicted sorted ISIs were plotted against
smoothing the binary signals to continuous signals, we estimated
those acquired by ϕ. These plots were used for visualizing which
the correlation coefficient, r, as a function of ˆ
σ. For each recording,
ISIs of ϕ were well captured by the model and which were not.
r was averaged over 9 trials of simulations lasting 1 s each.
Such comparisons are especially useful in testing the ability of the
The selection of an optimal time-scale for the time-dependent
model to predict characteristics of an STN neuron such as the onset
metrics is not a trivial task as it results from the interplay of
and the recession of a bursting spike train. To compare y's rhythm,
many different factors such as the distribution of the spikes in
Ry, with ϕ's rhythm, Rϕ, a bin width was estimated using Freed-
the MERs and the degree of similarity between the predicted and
man Diaconis rule For all MERs, we
recorded spikes (in analogy to Herein, we
calculated the h = 2 IQR(x) , where h is the bin width, IQR is the
propose single-valued metrics derived from the V–P metric that
inter-quartile range of the data x and n is the number of data sam-
‘‘integrate'' the quality of a prediction. For each real recording,
ples and we selected the median width (50 ms) as a universal bin
we estimate the V–P distance between the real spikes and those
width. Although these methods are preferable in applications to
of the prediction, V–Ppredict, as well as between the real spikes
real data for which there is no validated knowledge about the rele-
and those of a random spike train, V–Prandom. The random spike
vant time scales, they require visual validation. More importantly,
train had the same number of spikes and duration with those of
they do not allow for the functional characterization and precision
the real spike train with its spikes uniformly distributed across
analysis offered by the time-dependent methods.
the timespan of the real recording. The intersections between
Consequently, to give an objective and comparable estimate
V–Ppredict and V–Prandom defined the range of the time scales for
of neuronal variability, we used 2 time-scale depended measures
which the prediction could not be regarded as random For
of spike train (dis)similarity, namely the Victor–Purpura (V–P)
each V–P metric, there are two time-scale related points: a Spike
distance and the Schreiber et al. similarity measure. One of
Coincidence (SC) point below which any spike costs less if it is
the main arguments for using such measures is their potential
deleted/inserted than shifted and a Spike Rate (SR) point above
insight into the precision of the neuronal code
which the V–P metric is as low as possible Yet, a poor
The V–P distance
prediction can still result to a very small (large) SC (SR) point; e.g.
between two spike trains is
when a neuron predicts to fire randomly with a rhythm equal to
calculated by minimizing the cost of transforming one spike train
the average rhythm of the real recording. That is why we propose
into the other by means of 3 basic operations: (a) spike deletion
the Prediction Area (PA) between the two V–P metric curves as
(cost 1); (b) spike addition (cost 1); and (c) spike shift by an interval
an estimator of the overall quality of the prediction In
δt (cost q δt ). The cost per time unit, q, sets the time scale of the
particular, we calculated the ratio of the two areas below the
analysis. As q → 0 (no cost) the distance is equal to the difference
curves, RoA = Areapredict/Arearandom. An accurate prediction will
in the number of spikes. As q → ∞, it is cheaper to delete and
increase the area between the two curves and hence will make
create spikes than to shift a spike and so the distance approaches
RoA < 1. Please note that an RoA also gives an indication of a bad
the number of non-coincident spikes. Thus, by increasing the cost,
rate prediction: when the average predicted rhythm is not equal to
the V–P distance gradually transforms from a rate distance to a
the real one, the lower asymptote of V–Ppredict is y = x, with x > 0
temporal distance. Roughly speaking, 2/q is the time-difference
and that can make RoA > 1.
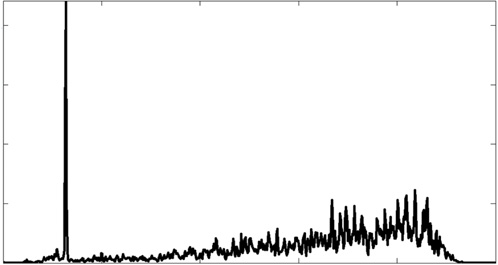
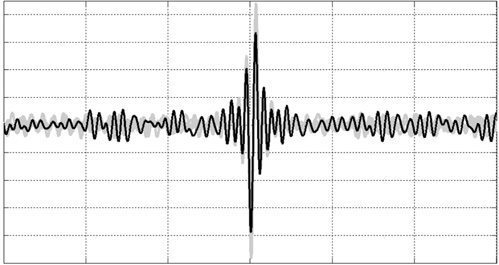
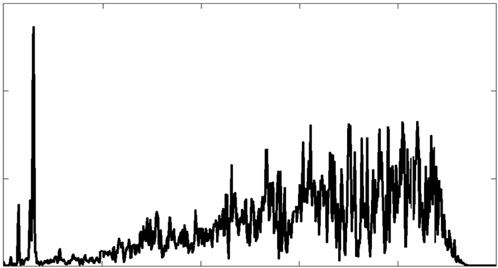
K.P. Michmizos et al. / Neural Networks 36 (2012) 146–156
ed LFP (SDU) -0.2
Fig. 3. Spike–LFP relationship for electrodes positioned inside the STN of 2 patients (a) STA-LFP. Amplitude is in standard deviation (SD) units. Superimposed (thick grey
lines) are the STAs computed for all LFPs from the same nucleus. (b) PSD estimate via Welch's method for the LFP on its left. (c) STA-LFP from a different patient. SD of the
resulting STA was calculated as in B. Superimposed (thick grey lines) are the STAs computed as in A. (d) Same as B.
3. Results
and 0.3, respectively. A typical value of w = 120 samples andp = 0.5 was used throughout the simulations in this study. For
Computer simulations were performed to evaluate the identi-
the LFPs, power spectral density (PSD) was also estimated using
fication methodology and the validation metrics. Thirty one MERs,
the Welch's method. LFPs were divided into 8 sections with 50%
acquired from the sensorimotor areas of 5 STN (3 patients), were
overlap, each section was windowed with a Hamming window
and the modified periodograms were averaged. PSDs of the MERsunder study revealed that there is a characteristic – for PD – beta
3.1. Spike triggered average of the STN-LFP
band peak and (d)) in the LFPs. Such a peak was absent inrecordings acquired from outside the STN borders (p < 0.05).
Local neuronal synchronization was determined using the
spike-triggered average (STA) of the LFP. The relationship between
3.2. Single neuron spike prediction
STN spike activity and the underlying LFPs is visualized in The processing of MERs, acquired from 2 subjects, is plotted. Data
Validation results after each step of the identification process
in upper figures received from Subject 2, left STN, central electrode,
are shown in for a single neuron MER. Data received from
1 mm below the theoretical target. Data in bottom figures received
Subject 3, left STN, lateral electrode, 1.5 mm below theoretical tar-
from Subject 3, left STN, lateral electrode, 1.5 mm below theoretical
get. Seven LFP-spike pairs, with w = 120 samples and p = 0.5,
target. For each spike, we obtained the LFP segment for a short time
were recruited as the training data. Models were validated with re-
window (±0.15 s lag) around that spike and then we averaged over
spect to the mean rhythm mean squared error, MSE, and 2 metrics,
those LFP segments. For significance levels, ISIs were randomized
namely the r and the RoA (see Section A 3rd order polyno-
and the SD of the resulting STA was calculated. Any components
mial was fitted to the data in a least squares sense and overlaid on
of the LFP that were not consistently phase locked to the spikes
each plot. The general trend of the parameterization of the LTI sys-
averaged out and are not visible in the STA. If spike times had
tem, for each MER, could then be assumed. In this example, models
no temporal relation to the LFP activity of surrounding neurons,
with nb < nf resulted in a poor spike prediction. On the contrary,
fluctuations in the LFP would average out during STA compilation,
models with M2 , n
resulting in a flat STA. The STA-LFP fluctuation indicates that spike
≤ 2 predicted the spike activity with great
times have a reliable temporal relation to the LFP. In and
accuracy (MSE < 0.75, r ≥ 0.8 and RoAs ≤ 0.6). Other models,
(c), one notes a sharp negativity at spike position (at zero lag)
e.g. M3, took advantage of the refinement process and stabilized
and a prominent upswing for negative and positive time lags, i.e.,
their performance near a constant value for each metric. This was
right before and after a spike occurred. The negative spike in the
also observed in most of the models in which nb + nf ≥ 3. That
STA as a response to spikes was common among electrodes and
being said, an over-parameterized model resulted in a poor prog-
patients. The presence of the zero-lag peak in all recordings is an
nosis (e.g. M3 and M3). Thus, to avoid overfitting the model to the
indication of a linear relation between spikes and LFPs, since the
training data, we kept the smallest possible parameter values that
STA is equivalent to computing the cross-correlation between the
gave good results. In the case presented here, one of the M2 models
binary output and the input (LFP) (for a proof see
was selected as the optimal model.
The STA fluctuation
Four of the sequentially estimated M2 models, shown in
duration (<0.02 s) and the zero lag STA peak restricted w to be
are presented in A visual comparison of the prediction
less than 240 samples and p equal to 0.5, respectively. But in
for one of them (the ‘‘third'' M2) reveals the model's ability to
practice, w and p were found sufficient to be as low as 90 samples
predict the overall structure of the spike train The
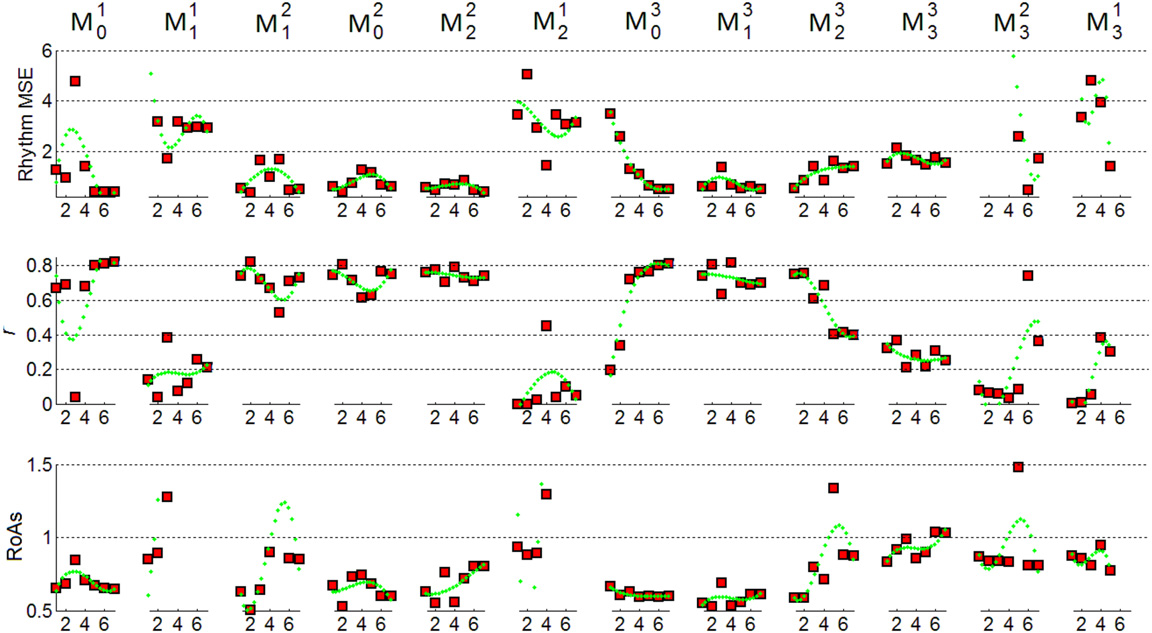
K.P. Michmizos et al. / Neural Networks 36 (2012) 146–156
Fig. 4. Identification procedure for single neuron MER. M b
symbolizes a H–W model with a linear component of n
b zeros and (nf + 1) poles. For each model, the same 7
training LFP-spike pairs were used, in the same order.
Fig. 5. Modeling example (a)–(c) 4M2 models, identified for the first, second, third and fifth training pairs. (d) The raw MER (up) and the second M2 model's prediction
(bottom). (e) The general, time-independent validation metrics: Rhythm estimation (up), CDF plot (middle) and sorted ISI plot (bottom). (f) The time-dependent metrics:Correlation coefficient of smoothed versions of y and ϕ (up) and V–P distance between y and ϕ with respect to time scale (bottom).
exact onsets and offsets of the bursts were somewhat inaccurate in
model's ability to detect the presence of a spike after an extended
the prediction. Nonetheless, even smaller bursts and single spikes
absence of spikes is somehow limited. This means that the model
were closely predicted, although no clear mark in the LFP signal
might not be totally accurate in predicting single spikes, especially
could be seen with the naked eye. There were also occasions
when the neuron is firing after a quiescent epoch. However, this
where spikes were simply missed (7.10–7.20 s) or fabricated
was not always the case, since 12 of 16 single-neuron models
(3.25–3.35 s). The model was able to predict the presence of both
predicted the ISI accurately (MSE-ISI was lower than 0.65 ms).
lonely spikes and bursts with great accuracy. The great accuracy
The RoA was estimated equal to 0.58 and r approached
of the model was reflected in the general, time-independent
the asymptote of y = 0.8 at a time resolution of 4 ms; the V–P
comparison using the estimated rhythms, the CDFs and the ISIs
metric remained very low (<0.2) until a temporal resolution of
The CDF plot showed that the model displayed a lack
6 ms and the SC point was found at a temporal resolution of 1 ms
of fit only for the last second. Nevertheless, model values lie in
(12 samples).
the border of the 95% confidence bounds. The maximum distance
The accuracy of the model to predict the spikes using LFPs
between model values and the 45° line was 7 spikes (at 7.55 s). A
recorded from the same electrode was also proven true when using
similar insufficiency of the optimum models was found in 4 of 16
LFPs acquired from adjacent electrodes. In the V–P distance
single-neuron models. An observable small discrepancy between
for predictions for two spike trains is shown. The predictions were
the large values of ISIs means that that the particular
made by optimal M2 models driven by LFPs from posterior and
K.P. Michmizos et al. / Neural Networks 36 (2012) 146–156
Fig. 6. Prediction of spikes using LFPs acquired from adjacent electrodes for (a) 1-neuron, and (b) 2-neuron MERs. Spikes received from central electrode for both cases. For
the prediction, the LFPs from Central, Posterior and Anterior electrodes were used. (left). Periodogram estimation for each of the LFPs used. (right) The V–P distance metric
with respect to the time scale, for each of the predictions.
anterior electrodes whereas spikes were acquired from the central
electrode. The raw recordings from adjacent microelectrodes were
Single-neuron models estimated from MERs received from five nuclei (threepatients). Numerator vector B = [b
spike-free as the microelectrodes were not close enough to any
1 , b2, . . , bk] and denominator vector F
[f1, f2, . . , fk] represent the polynomial coefficients of the numerator and the
neuron. All LFPs were acquired inside the STN. For both cases,
denominator of the LTI system, respectively.
the posterior electrode was placed inside the sensorimotor area
Hemisphere Recording
LTI module [Numerator]
(beta band peak present) but the anterior electrode was not (as
identifier [Denumerator]
indicated by the presence of alpha rhythm and the absence of
beta band energy). The first order statistics of the impedances
[0, 1] [1, −0.649]
of the microelectrodes used in this study are µ = 1447 k and
σ = 52 k. Hence the interfering input (i.e. the input for which the
[1, −1.764, 0.781]
microelectrodes were unintentionally responsive) was not altered.
−0.5 (Posterior)
[0, 1] [1, −0.956]
Yet, when the LFP recording electrode is 2 mm away from the spike
[0, 1] [1, −0.451]
[0, 1] [1, −0.987]
recording electrode, the modifying input is expected to increase.
The accuracy of the prediction was lowered moderately when LFPs
[0, 1] [1, −0.068]
[0, 1] [1, 0.866]
from other electrodes were used, compared to the accuracy of
−1.0 (Posterior)
[0, 1] [1, −0.949]
the model estimated from the same electrode Specifically,
[0, 1] [1, −0.279]
for the first prediction, the RoA increased from 0.80 (center LFP-
[0, 1] [1, −0.871]
driven model) to 0.89 and 0.90 for posterior and anterior LFP-
[0, −0.952, 1] [1, 0.859]
driven models, respectively. For the second prediction, the RoA
[0, 0.962, 1] [1]
increased from 0.87 (center-LFP driven model) to 0.91 and 0.92 for
[0, −0.999, 1] [1]
posterior and anterior LFP-driven models, respectively.
−1.5 (Posterior)
[0, −40.970, 1] [1]
Cumulative results with models estimated from single-neuron
[0, −0.523, 1] [1]
MERs help to establish the validity of the identification procedure.
[0, −1.075, 1] [1, −0.979]
Each model was estimated and validated separately Themodel's predictability was a function of both the quality of MER
By looking at the SC and SR points, we verified that models
(observability of the spikes) and the validation metrics used.
estimated from single neuron MERs predicted the spike rhythmbetter than those estimated from 2-neuron recordings. The last
3.3. Multi-neuron spikes prediction
were slightly better compared to the models estimated from3-neuron recordings. The average SC point for single neuron
The identification scheme was also tested and found to be
predictions was found at 0.57 ms (±0.12 ms) whereas that for
effective in MERs exhibiting spikes received from an ‘‘area'' of
double and triple neuron MER predictions was found at 0.17 ms
neurons. A typical 2-neuron MER might have high amplitude
(±0.24 ms) and 0.05 ms (±0.17 ms), respectively. The average SR
spikes received from a burster neuron very close to the electrode
point for single neuron predictions was found at 5.0 s (±0.5 s),
and low amplitude spikes received from a farther apart neuron. A
whereas that for double and triple neuron MER predictions was
comparison between the V–P distance for optimal single-neuron
found at 1.0 s (±0.5 s) and 0.8 s (±0.3 s), respectively. The rhythm
and multiple neuron models is shown in For a temporal
predictability of the models can be verified by looking at the V–P
resolution ranging between 2.9 ms and 112 ms, the V–P distance
distance for large time scales (>1 s). All (16) single-neuron, 7 out
in single-neuron MERs predictions was significantly lower than
of 10 2-neuron and 4 out of 5 3-neuron models predicted the
the V–P distance for 2 or 3-neuron MERs (two-sample t-test, p <
rhythm very accurately (V–P distance <0.1). The remaining models
predicted the rhythm with V–P distance = 0.2 (±0.07).
K.P. Michmizos et al. / Neural Networks 36 (2012) 146–156
approaches in which simple linear interaction between LFP andspike signals is tested (e.g., with means of coherence or correlationthe approach used in our study exploited thepattern of the LFP in a nonlinear way to infer spike activity.
A reasonable argument against our proposed modeling ap-
proach is that spikes could be mainly predicted by a low-frequencycomponent remaining in the LFP after low-pass filtering the spik-ing signal. Firstly, if the spike predictions were due to low-frequency ‘‘spike-remains'', one could not use the LFPs fromelectrode-j to predict the spikes from an electrode-i (i ̸= j), asshown in Secondly, other models that, unlike the H–Wmodel, are not data-driven (e.g. the Izhikevich model and the bi-exponential model were also successful in predicting the spikes fromLFPs acquired from MERs in the same data set. These models do
Cumulative V–P distance for single-neuron and multi-neuron models.
not rely on the input–output relationship as the H–W model does.
Normalized spike distance metric is shown. The grey area indicates the time
Thirdly, other researchers
resolution for which there is a significantly different amplitude between V–Pdistance metric for single neuron models and double (triple) multi neuron models.
have tried a different approach to prove that the pre-
Black lines indicate average V–P distance.
diction is not due to a spike artifact: they excluded the 1 ms areaaround a spike and they interpolated the LFP with a sample-signal
3.4. Model residuals distribution
found close to each spike. They concluded that model performancewas superior when spikes were not removed. As our signals' energy
By looking at the probability density function of the residuals,
was located in the >100 Hz area (and hence our prediction relied
one can tell whether the assumptions made were reasonable and
on the fast changes in the LFP signal) and we aimed for the best
if the choice of model was appropriate. Residuals can be thought
possible model, we did not follow this approach.
of as elements of variation unexplained by the fitted model. If
Whether the spike prediction is due to physiological mech-
we ensure that a model is able to simulate the neuronal spike
anisms or to pathophysiological changes in the STN cells mer-
rhythm with a reasonable accuracy, the residual rhythm Ry − Rϕ
its further clarification. Spikes are predicted by cortical LFPs in
must follow a Gaussian distribution. In cumulative results,
healthy animal models From a neurophysiolog-
acquired for all our estimated models of 1-, 2 and 3-neuron MERs
ical point of view, the STN is a ‘‘close'' nucleus (please see Section
are shown. On each probability histogram, we superimpose the
2.A from Hence, it seems reasonable to say
corresponding theoretical distribution having first order statistics
that the general formulation is related to the physiology of the STN
that were estimated from the raw residuals. Gaussian distribution
neurons and not to PD. Whether our current modeling scheme can
of residuals was common among recordings and nuclei for bin
be expanded to other neurons (e.g. in Vim and SNr) requires more
widths <50 ms. This is theoretically justified since it is known that
clinical data than those we currently have.
a superposition of Gaussian distribution signals results in a signal
The good predictability of our models may be, at least partly,
having the same (Gaussian) distribution.
attributed to the existence of coincidence-detector neurons foundinside the STN By comparing the
STA-LFPs, one can deduce if two neurons respond to the same inputwith the same response (spike). No two STA-LFPs were identical,
Our simulation results confirm that the proposed identification
yet they all had a common pattern: a deep low oscillation very
scheme, guided by a combination of spike-timing validation
close to when the spike occurred followed and preceded by high-
metrics, succeeded in finding a data-driven model of the neural
rising waves (see Such STA-LFP oscillations were visible only
area inside the STN for all 31 MERs in our data set. Unlike other
in the electrode from which we acquired the spikes. Oscillations
Fig. 8. Cumulative density estimation for residuals of the estimation of the spike rhythm for all models. The theoretical Gaussian distribution with mean and SD equal to
the mean and SD of the residual signal, respectively, is superimposed in each graph.
K.P. Michmizos et al. / Neural Networks 36 (2012) 146–156
were almost identical in frequency but not in amplitude, across
and the ISI plot with a single-valued metric. That being said, an
MERs. The amplitude variation might depend on both the
accurate CDF plot and a somewhat accurate ISI plot could still be
electrode–neuron distance and the neuron characteristics. If it was
accompanied with a bad (close to 1) RoA value, resulting from a
only due to the electrode–neuron distance, the STA-amplitude
poor prognosis for the model. That was the case when the predicted
would follow the spikes' amplitude which was not the case in our
spike rhythm was constant and similar to the mean true spike
data set. Hence it seems safe to assume that the LFP-oscillation
rhythm. On the other hand, a good (less than 1) RoA value could
would increase in amplitude under the presence of two neurons
point out an accurate prediction but failed to describe a model with
firing simultaneously. Since the LFP signal-to-noise ratio would be
mediocre performance, since it missed the information of when the
higher, one would expect a better prediction for the neural activity.
model failed to predict correctly the spikes.
Our analysis of the STA-LFP also revealed a deviation of the STA
In addition, spike timing accuracy of the models' prediction falls
fluctuation duration compared to STAs in previous studies. The
with the number of neurons recorded. Training of models with
elevated power at beta frequencies (13–35 Hz) of the LFPs received
single neuron MERs resulted in very accurate spike predictions.
inside the dorsolateral (motor) area of the Parkinsonian STN at
Note that a value of Roa < 0.6 (observed in means, on
rest (for a discussion see
average, that 90% of the recorded spikes were predicted by the
possibly explain those differences in the STA-LFP fluctuation
model (with a ±25 sample distance) and at the same time 90%
duration, compared to previous STA-LFP analyses. In addition, high
of the predicted spikes were also existent in the raw data (with
frequency oscillations (>150 Hz), only present in patients with
a ±25 samples distance); this is what we define as a 90% accuracy
for the model. The means the RoA for models trained and validated
were also observable in all MERs (p < 0.01)
with single neuron MERs (16 in total) was 0.70; that corresponds
(see and (d)). Consequently, the low frequencies (<2 Hz)
to models with 80% accuracy, on average. Results are in agreement
did not dominate the signal; rather the slow oscillations were
with two, unrelated to each other, modeling approaches that
modulated in the high amplitude beta (or higher) oscillations.
exploit the LFPs to predict the STN spikes (see
Hence, the spectral characteristics of the STN-LFP signals were
For multi-neuron MERs (15
different from the ones estimated from the S1
in total), the mean RoA increases to 0.8 for 2-neuron MERs (>61%
and the V1 cortical areas. This may explain
accuracy) and 0.85 for 3-neuron MERs (>53% accuracy). However,
the difference in fluctuation duration between the estimated STA
this significant decrease of temporal accuracy in spike predictionmay not only be due to a mediocre model performance. In multi-
and (c)) and other STA-LFPs already presented (e.g., Figs.
neuron MERs, some of the spikes of the farthest recorded neuron
1 and 2 of and Fig. 2 of Our
are unavoidably covered by the noise level. In a visual observation
hypothesis is further supported by studies estimating the STA from
of a multi-neuron MER, one could recognize spikes inside the noise
high-pass filtered versions of S1 LFPs (see Fig. 4 of
level; although their amplitude was smaller than the noise level,
its shape was clearly observable. That was not the case for single
By training the H–W models with small LFP segments around
neuron MERs where even a significant decrease of the amplitude
each spike, we aimed at capturing the high frequency component
of a spike (usually as a consequence of a bursting firing of that
of the LFP. We chose to train the data with small segments of LFPs
neuron) would still result in a spike amplitude that would be well-
because (a) most of the energy of our signals was concentrated in
distinguishable from the noise level. Indeed, for most (13 out of
the >100 Hz area and in the β-band area and (b) mul-
15) of the multi-neuron MERs, the resulted model's residuals had
tiple small segments allow the refinement of models with spike
a larger predicted rhythm than the real rhythm. This argument
prediction reaching a plateau fast Oscillations with fre-
is further supported by the estimated PDF of the residuals for
quencies equal or lower than β-band were not captured by the
3-neuron recordings the number of misdetected spikes in
window. That was done on purpose as (a) β-energy was always
the right lobe of the Gaussian (over-prediction) was significantly
present during the recording (the β-band activity was a prerequi-
larger compared to the one in the left lobe. This means that the
site to include a MER in our data set) and (b) low frequency com-
3-neuron models either over-predicted the recorded activity or
ponents are much more likely traveling from farther neurons (just
they predicted some of the spikes that corresponded to recorded
like one can only hear the low frequencies of a song played in a
spikes lost inside the MER noise.
radio player far away; also see
Consequently, millisecond precision, shown to be used for
By restricting ourselves to high frequencies, we minimize the mod-
temporal coding was not totally safe
ifying noise arriving from farther neurons (the spiking activity of
to be inferred from LFPs in multi-neuron MERs. But even in those
which we do not record) and we focus on the synaptic activity of
recordings, we can still assume that, irrespective of whether they
the nearest to the electrode neuron(s).
are important for coding, temporal aspects of neural spiking used
However, the strengths of modeling are tempered by the
for rate coding or coding on spike timing on the scale of 50–150 ms
necessary simplifications made in any reasonable model. In our
can be moderately well inferred from the LFPs. This is very
case, the model does not explicitly take into consideration the
crucial since electrophysiological research in the last 25 years has
existence of glutamatergic synapses between STN neurons, which
provided evidence that individual impulses in the impulse trains
have been strongly suggested
from each neural cell may sometimes be quite precisely timed
even if still debated. But still, the feedback
and hence of great importance. For instance, impulse trains from
from an STN neuron to one that was being recorded, was implicitly
single neurons may show above-chance recurrences of exactly
embedded into the model through the LFPs.
timed sequences of several impulses
Not a single validation metric was found adequate to fully
Moreover, when impulse trains from different neurons recorded
characterize the quality of a prediction. For example, the metric
at the same time are compared, there may be above chance
r, by itself, was not always informative of the quality of the
repetitions of exactly timed impulse patterns shared between the
prediction. The r depended heavily on the rhythm since a binary
different trains. Furthermore, there is increasing evidence that
signal with low number of spikes per second has large ‘‘0'' areas. In
such exact timing of impulses correlates with behavior
these signals, even if the spikes were heavily misdetected, r would
or with sensory analysis and there
still be large. Such a problem was often revealed by estimating the
is increasing acceptance that exactly timed patterns of impulses
ISIs That is why, to decide on the optimal model, one
may be of general significance in the information processing
had to consider a combination of validation metrics such as a CDF
accomplished by the brain.
K.P. Michmizos et al. / Neural Networks 36 (2012) 146–156
Ultimately, the model may represent a first step in realizing
model able to predict the spikes for a specific electrode, nucleus
neural prostheses to replace damaged subregions of the basal
or patient was found. Rather, we estimated a MER-specific model.
ganglia that could initiate and control a movement using a brain
Hence, the strong dependence of the model parameters (and
computer interface. This is in analogy to various central nervous
accordingly of spike predictability) on electrode position suggests
system neural prostheses currently under development and
that the accuracy of such predictions does depend on the recording
application. An example is a research study that aims to replace a
site inside the STN. Our proposed modeling identification scheme
brain region (hippocampus) with a cognitive prosthesis
might enable the design of time-adaptive DBS stimulation that can
That being said, achieving a clinically viable model that can
be tailored to the needs of each individual PD patient. However to
predict the electrophysiological function of a region – the motor
reach this goal and test the DBS effects on the firing activity, the
area in our case – of STN requires more than the H–W model shown
model input must be modified accordingly.
here. The model presented in this paper is meant primarily todemonstrate the methodology and the feasibility for capturing thespike timings and the rhythm of a small ensemble of STN neurons.
Although replacing higher-thought processes such as planning
or execution of motor functions is not an easy target for our model,
Albin, R., Young, A., & Penny, J. (1989). Functional anatomy of the basal ganglia.
other possible extensions seem far more reachable. The models
Trends in Neurosciences, 12, 366–375.
presented in this paper could allow analysis of the effects of stim-
Alexander, G. E., & Crutcher, M. D. (1990). Functional architecture of basal ganglia
ulation on the spike activity of the neurons that surround the elec-
circuits: neural substrates of parallel processing. Trends in Neurosciences, 13,
trode with and without DBS signal, something difficult to achieve
experimentally. In other words, they can be used as the test bed for
Alexander, G. E., Crutcher, M. D., & DeLong, M. (1990). Basal ganglia—
thalamocortical circuits: parallel substrates for motor, oculomotor, prefrontal
current and future theories on the STN and even shed some light on
and limbic functions. In H. Uylings, C. Van Eden, J. De Bruin, M. Corner, &
the effect that the DBS has on the STN function. One can allow for a
M. Freenstra (Eds.), Progress in Brain Research, Vol. 85 (pp. 119–146). Oxford:
physiologically justified alternation of the model's input (LFP) and
compare the output with certain known physiological or patholog-
Bedard, C., Kröger, H., & Destexhe, A. (1986). Modeling extracellular field potentials
and the frequency-filtering properties of extracellular space. Biophysical Journal,
ical patterns of neural behavior. For example, it has been shown
that STN DBS causes a significant attenuation in power in the beta
Bedard, C., Kröger, H., & Destexhe, A. (2004). Modeling extracellular field potentials
band of the LFPs at rest A recent study has
and the frequency-filtering properties of extracellular space. Biophysical Journal,
also been conducted on the combined effect that levodopa and on-
going DBS have on the beta band of the LFPs recorded from the STN
Bedard, C., Kröger, H., & Destexhe, A. (2006). Model of low-pass filtering of local field
potentials in brain tissue. Physical Review, 73, 051911.
of PD patients By changing appropriately
Bergman, H., Wichmann, T., Karmon, B., & DeLong, M. (1994). The primate
the model's input, different theories of the DBS effect on the LFP
subthalamic nucleus, II. Neuronal activity in the MPTP model of parkinsonism.
can be tested. The inferred spike activity could then be compared
Journal of Neurophysiology, 72, 507–520.
with known patterns to assess the effect of each stimulation pat-
Bevan, M., Magill, P., Termanc, D., Bolamb, J., & Wilsond, C. (2002). Move to
tern on the neuronal activity of the modeled neural area.
the rhythm: oscillations in the subthalamic nucleus—external globus pallidusnetwork. Trends in Neurosciences, 25, 525–531.
Finally, our analysis of the ability of the model to predict spikes
Buzsáki, G. (2006). Rhythms of the brain. Oxford, UK: Oxford University Press.
using LFPs acquired from adjacent electrodes is somewhat limited
Chicharro, D., Kreuz, T., & Andrzejak, R. G. (2011). What can spike train distances
by the array of microelectrodes currently used. By building a MER-
tell us about the neural code? Journal of Neuroscience Methods, 199, 146–165.
specific model, one can simulate the neural activity in a given depth
Danish, S., Moyer, J., Finkel, L., Baltuch, G., Jaggi, J., Priori, A., et al. (2007). High-
inside the STN. This approach is only limited by the number of
frequency oscillations (>200Hz) in the human non-Parkinsonian subthalamicnucleus. Brain Research Bulletin, 74, 84–90.
the recording microelectrodes inside the STN. A more expanded
Dayhoff, J. E., & Gerstein, G. L. (1983). Favored patterns in spike trains: II. Application
array of models will raise interesting questions concerning the
Journal of Neurophysiology, 49, 1349–1363.
effect that the input (LFP) recorded from adjacent electrodes has
Eccles, C. (1951). Interpretation of action potentials evoked in the cerebral cortex.
on the predicted spike activity, received from a single electrode.
Journal of Neurophysiology, 3, 449–464.
It has been shown that the extracellular space behaves like an RC
Farries, M. A., Kita, H., & Wilson, C. J. (2010). Dynamic spike threshold and zero
(lowpass) filter for the LFPs
membrane slope conductance shape the response of subthalamic neurons tocortical input. Journal of Neurophysiology, 30(39), 13180–13191.
Hence, it should be interesting to work on the interconnection
Freedman, D., & Diaconis, P. (1981). On the histogram as a density estimator: L2
among the various models in a certain depth by expanding
theory. Probability Theory and Related Fields, 57, 453–476.
the current models to receive multiple inputs and projecting
Giannicola, G., Marceglia, S., Rossi, L., Mrakic-Sposta, S., Rampini, P., Tamma, F.,
to single (MISO) or even multiple (MIMO) outputs. In addition,
et al. (2010). The effects of levodopa and ongoing deep brain stimulation on
since the formation of the microelectrodes already records the
subthalamic beta oscillations in Parkinson's disease. Experimental Neurology,226, 120–127.
neural activity in various depths, this array of models can also be
Gillies, A. (1996). Electrical properties of subthalamic nucleus projection neurons.
expanded to 3D or even 4D space. If the simultaneous recording
In J. Bower (Ed.), Computational neuroscience (pp. 65–70). New York: Academic
at various depths inside the STN becomes technologically feasible
and medically justifiable, such neurophysiological models of the
Gillies, A., & Willshaw, D. (1998). A massively connected subthalamic nucleus leads
to the generation of widespread pulses. Proceedings of the Royal Society of London
intranuclear spike activity would help to shed some light on the
Series B, 265, 2101–2109.
physiological and pathological properties of the STN.
Hikosaka, O. (1991). Basal ganglia: a possible role in motor conditioning and
learning. Current Opinion in Neurobiology, 1, 638–643.
Hildebrand, F. B. (1956). Introduction to numerical analysis. New York: McGraw-Hill
(pp. 319–323).
Iwahori, N. (1978). A Golgi study on the subthalamic nucleus of the cat. The Journal
Information is coded in the population activity of neurons,
of Comparative Neurology, 182, 383–398.
especially in sensory, motor and other higher cortical brain regions.
Kane, A., Hutchison, A. W., Hodaie, M., Lozano, A., & Dostrovsky, J. (2009). Dopamine-
Any neuron responds primarily to a combination of chemical and
dependent high-frequency oscillatory activity in thalamus and subthalamic
electrical signals, some of which are believed to influence (if not
nucleus of patients with Parkinson's disease. NeuroReport, 20, 1549–1553.
dominate) the LFP. Because the mechanisms underlying neural
Kass, J. I., & Mintz, I. M. (2006). Silent plateau potentials, rhythmic bursts,
and pacemaker firing: three patterns of activity that coexist in quadristable
firings are inherently nonlinear, our modeling approach required
subthalamic neurons. Proceedings of the National Academy of Sciences of the
methods from nonlinear system identification theory. No global
United States of America, 103, 183–188.
K.P. Michmizos et al. / Neural Networks 36 (2012) 146–156
Kita, H., Chang, H., & Kitai, S. (1983). The morphology of intracellularly labeled rat
Michmizos, K. P., Tagaris, G., Sakas, D., & Nikita, K. S. (2009b). Employing LFPs
subthalamic neurons: a light microscope analysis. The Journal of Comparative
and spikes to model the non-linear behavior of the STN. BMC Neuroscience, 10.
Neurology, 215, 245–257.
Kostoglou, K., Michmizos, K. P., Stathis, P., Sakas, D., Nikita, K. S., & Mitsis, G.
Mitzdorf, U. (1987). Properties of the evoked potential generators: current source-
(2012). Prediction of the Parkinsonian subthalamic nucleus spike activity from
density analysis of visually evoked potentials in the cat cortex. International
local field potentials using nonlinear dynamic models. In IEEE BIBE'12. Larnaca,
Journal of Neuroscience, 33, 33–59.
Okun, M., Naim, A., & Lampl, I. (2010). The subthreshold relation between cortical
Kreuz, T., Haas, J. S., Morelli, A., Abarbanel, I., & Politi, A. (2007). Measuring spike
local field potential and neuronal firing unveiled by intracellular recordings in
train synchrony. Journal of Neuroscience Methods, 165, 151–161.
awake rats. Journal of Neuroscience, 30, 4440–4448.
LeCun, Y., Bottou, L., Orr, G., & Muller, K. (1998). Efficient BackProp. In G. Orr, &
Prescott, T., Gurney, K., Montes-Gonzalez, F., Humphries, M., & Redgrave, P. (2002).
K. Muller (Eds.), Neural networks: tricks of the trade. New York: Springer.
The robot basal ganglia: action selection by an embedded model of the basal
Levenberg, K. (1944). A method for the solution of certain non-linear problems in
ganglia. In L. Nicholson, & R. Faull (Eds.), The basal ganglia, vol. VII (pp. 349–358).
least squares. The Quarterly of Applied Mathematics, 2, 164–168.
New York: Kluwer Academic.
Lewicki, M. S. (1998). A review of methods for spike sorting: the detection
Quiroga, R. Quian, Kreuz, T., & Grassberger, P. (2002). Event synchronization: a
and classification of neural action potentials. Network: Computation in Neural
simple and fast method to measure synchronicity and time delay patterns.
Systems, 9, 53–78.
Physical Review E, 66, 041904.
Ljung, L. (1999). System identification theory for the user. New Jersey: Prentice Hall
(pp. 327–329).
Rasch, M., Gretton, A., Murayama, Y., Maass, W., & Logothetis, N. (2008).
Ljung, L., & Söderström, T. (1986). Theory and practice of recursive identification.
Inferring spike trains from local field potentials. Journal of Neurophysiology, 99,
Cambridge, MA: MIT Press (Chapter 5).
Logothetis, N. K. (2008). What we can do and what we cannot do with fMRI. Nature,
Rasch, M., Logothetis, N., & Kreiman, G. (2009). From neurons to circuits: linear
estimation of local field potentials. Journal of Neurophysiology, 29, 13785–13796.
Mainen, Z., & Sejnowski, T. (1995). Reliability of spike timing in neocortical neurons.
Rieke, F., Warland, D., de Ruyter van Steveninck, R., & Bialek, W. (1997). Spikes
Science, 268, 1503–1505.
exploring the neural code. Cambridge, MA: MIT Press (pp. 289–295).
Marquardt, D. (1963). An algorithm for least-squares estimation of nonlinear
Schreiber, S., Fellous, J. M., Whitmer, J. H., Tiesinga, P. H. E., & Sejnowski, T. J. (2003).
parameters. SIAM Journal on Applied Mathematics, 11, 431–441.
A new correlation based measure of spike timing reliability. Neurocomputing,
McClurkin, J. W., & Optican, L. M. (1996). Primate striate and prestriate
cortical neurons during discrimination. I. Simultaneous temporal encoding of
Schultz, W. (1998). Predictive reward signal of dopamine neurons. Journal of
information about color and pattern. Journal of Neurophysiology, 75, 481–495.
Neurophysiology, 80, 1–27.
Michmizos, K. P., & Nikita, K. S. (2010). Can we infer subthalamic nucleus spike
Shen, K. Z., & Johnson, S. W. (2003). Group II metabotropic glutamate receptor
trains from intranuclear local field potentials? In Proceedings of the 32rd IEEE
modulation of excitatory transmission in rat subthalamic nucleus. Journal of
conference on engineering in medicine and biology, EMBC. Buenos Aires, Argentina
Physiology.
(pp. 5476–5479).
Song, D., Chan, R. H., Marmarelis, V. Z., Hampson, R. E., Deadwyler, S. A., & Berger,
Michmizos, K. P., & Nikita, K. S. (2011a). Local field potential driven Izhikevich model
T. W. (2007). Nonlinear dynamic modeling of spike train transformations for
predicts a subthalamic nucleus neuron activity. In Proceedings of the 33rd IEEE
hippocampal–cortical prostheses. IEEE Transactions on Biomedical Engineering,
conference on engineering in medicine and biology, EMBC. Boston, USA, August
(pp. 5900–5903).
Tsirogiannis, G., Tagaris, A., Sakas, D., & Nikita, K. S. (2010). A population level
Michmizos, K. P., & Nikita, K. S. (2011b). Addition of deep brain stimulation
computational model of the basal ganglia that generates Parkinsonian local field
signal to a local field potential driven Izhikevich model masks the pathological
potential activity. Biological Cybernetics, 102, 155–176.
firing pattern of an STN neuron. In Proceedings of the 33rd IEEE conference on
Victor, J. D., & Purpura, K. P. (1996). Nature and precision of temporal coding in visual
engineering in medicine and biology, EMBC. Boston, USA (pp. 7290–7293).
cortex: a metric space analysis. Journal of Neurophysiology, 76, 1310–1326.
Michmizos, K. P., Papavasileiou, V., Sakas, D., & Nikita, K. S. (2010). Mathematical
Victor, J. D., & Purpura, K. P. (1997). Metric-space analysis of spike trains, algorithms
physiological modeling of the relation between local field potentials and
and application. Network: Computation and Neural Systems, 8, 127–164.
spikes recorded inside the subthalamic nucleus. In Proceedings of the 10th IEEE
Villa, A. E. P. (2000). Empirical evidence about temporal structure in multi-unit
conference on information technology and applications in biomedicine, ITAB. Corfu,Greece (pp. 1–4).
recordings. In R. Miller (Ed.), Conceptual advances in brain research series, Time
Michmizos, K. P., Sakas, D., & Nikita, K. S. (2012). Prediction of the timing and the
and the brain (pp. 1–51). London: Harwood Academic Publishers.
rhythm of the Parkinsonian subthalamic nucleus neural spikes using the local
Wingeier, B., Tcheng, T., Koop, M. M., Hill, B. C., Heit, G., & Bronte-Stewart, H. M.
field potentials. IEEE Trans. Inf. Technol. Biomed., 16(2), 190–197.
(2006). Intra-operative STN DBS attenuates the prominent beta rhythm in the
Michmizos, K. P., Tagaris, G., Sakas, D., & Nikita, K. S. (2009a). Towards input output
STN in Parkinson's disease. Experimental Neurology, 197, 244–251.
non-linear modeling of the subthalamic nucleus using intranuclear recordings.
Zanos, S., Zanos, T. P., Marmarelis, V. Z., Ojemann, G. A., & Fetz, E. E. (2012).
In Proceedings of the 4th IEEE/EMBS conference on neural engineering 2009.
Relationships between spike-free local field potentials and spike timing in
Antalya, Turkey (pp. 601–604).
human temporal cortex. Journal of Neurophysiology, 107(7), 1808–1821.
Source: http://biosim.ntua.gr/sites/default/files/Michmizos_NN2012.pdf
Informational Shocks, O-Label Prescribing and the Eects of Physician Detailing Bradley T. Shapiro∗ This Version September 1, 2015 (Preliminary Version. Comments Welcome.) Promotional strategies employed by pharmaceutical rms to convince physicians to prescribe their products are the subject of considerable regulatory scrutiny. In partic- ular, regulators worry that rms may use sales reps to try to convince physicians to
Hong Kong NAME OF THE MEDICINE Human prothrombin complex, powder for injection. DESCRIPTION Prothrombinex®-VF is a sterile freeze-dried powder containing purified human coagulation factors II, IX and X and low levels of factors V and VII. It is prepared from blood collected from voluntary donors. The concentrate is prepared by adsorption of coagulation factors from plasma onto an