Revisedryansusmita_may 20
Similarity in Network Structures for in vivo and in vitro Data from
the Japanese Toxicogenomics Project
Ryan Gill1, Somnath Datta2,
Susmita Datta
1 Department of Mathematics, University of Louisville, Louisville, KY 40292, USA2 Department of Bioinformatics and Biostatistics, University of Louisville, Louisville, KY40202, USA
1. Introduction We provide a partial answer to the important question in Toxicogenomics
whether in-vivo microarray expression data based on animal studies can be replaced by in-
vitro data. We consider the TGP dataset which contains over 21,000 arrays for rats treated
with mainly human drugs and profiled using the Affymetrix RAE230_2.0 GeneChip®. The
main target organ profiled is liver. In a previous study, Uehara et al. (2010) identified the
genes commonly up-regulated both
in vivo and
in vitro after treatment with three different
drugs clofibrate, WY-14643 and gemfibrozil. This study was one of the first to create an
in
vivo i
–
n vitro bridge for the validation of a genomic biomarker with those three compounds. In
this analysis, we try to provide a comprehensive view of the
in viv –
o in vitr
o bridging across all
the genes (probe sets) for all the 131 drugs provided in the challenge data. Moreover, ourapproach is not only to observe the similarities in gene expressions of individual genes but toidentify the similarities of the network connectivity of all the similar genes across all thechemicals. Methodologically, we consider this question from a statistical perspective and applya significance test to examine if there is a difference between the genomic networks for thetwo different types (
in vivo/
in vitr )
o after accounting for different dosages of the drugs, and
sacrifice times of the rats. In order to construct the networks of genes and then finding thedifferences/similarities of the networks for the two types we use the approach similar to theframework for differential network analysis described in our earlier work in Gill et al. (2010).
Construction of the networks for each type of data is based on a connectivity score measuringthe association between each pair of genes. We apply a connectivity score constructed using apartial least squares (PLS) method that captures the predictability of each gene's expressionfrom a pairing gene after adjusting for other genes and additional covariables (such as dosage)and thus extending our earlier approach to network and differential network analysis (Pihur etal., 2008; Gill et al., 2010; Gill et al., 2012).
In order to study the expression pattern and the network structures, important data
preprocessing is required to account for type, dose, and sacrifice time effects. There aresubstantial differences between the expression values of the MAS5 preprocessed data from the
in vivo and
in vitro samples and any naive attempt (such as a gene by gene -
> test) might find
that all genes are significantly differentially expressed in the two types. We build in theadditional preprocessing in our linear model (ANOVA) for log-gene expressions. Similarly,these effects are included in our model for the computation of the PLS scores for the networkanalysis. These are detailed in the next section.
2. Data We analyze part of the challenge dataset from the Japanese Toxicogenomics Project
and compare the MAS5 preprocessed data from the "single dose study
in vivo experiment
using Sparague-Dawley rats" with the "
in vitro study using hepatocytes from Sparague-
Dawley rats" for 131 drugs. The
in vivo dataset for each drug has microarray expression
values of 31099 genes for 48 rats at four different dose concentrations (control, low, middle,and high) and four different sampling times (3, 6, 9, and 24 hours) with three observations ateach combination of the levels for these factors. The in-vitro dataset for each drug hasmicroarray expression values of the same genes for 24 rats at four dose concentrations withthe same labels and three different sampling times (2, 8, and 24 hours) with two observationsat each combination of the levels. The possibility of using the FARM preprocessed data wasalso considered, but many of the drugs have many genes with expression value 0 for allobservations which precludes the use of regression or even correlation methods since there isno variation in the value of these variables.
3. Methods First, we used a nested ANOVA model to assess the effects of
TYPE (
in viv /
vitro), drug dose (
DOSE) , and sacrifice time (
SAC) on the expression levels of 31099 genesfor each drug. Specifically, for each drug the mean expression value for the 3th observation forthe 1th gene is modeled as
.31 œ
TYPE31 Ð
TYPE‡
SA ‡
Before fitting the ANOVA model we take the logarithm of the centered expression levels; thelogarithm of the expression values are centered with respect to all genes of the given type. Foreach drug, the p-values for
TYPE are computed for each gene under the assumption that theexpression values follow a normal distribution with homogeneous error variance. We usethese preliminary ANOVA analysis to determine the genes for which the expression are notsignificantly different for two different types (
in viv
o vs.
in vitr )
o at a pairwise type 1 error
rate of 0.05. Summarizing the results for all the drugs we find there are 473 genes for whichthe
TYPE effect is not significant for at least 80% of the drugs. In other words, the expressionprofiles of this common set of genes appear to be similar for many of the drugs. Thus, these473 genes can be taken as common bridging genes between
in viv
o and
in vitr
o studies across
a great majority of the drugs. However, as the genes do not work independently we want toconstruct the network of those genes and check their differential behavior across two types.
The tests described in this section are based on connectivity scores =35 which measures
the association between the t
3 h and 5th genes in a network. Our earlier methods (Gill et al.,
2010) for differential network connectivity are modified to allow for additional covariates. Weestimate the coefficients for these additional covariates at the same time that the coefficientsused to compute the connectivity scores are obtained. Let B3 be the centered and scaled 8-dimensional expression vector for the t
3 h gene. The method of computing the PLS scores that
is described in Pihur et al. (2008) uses separate PLS models B
4Á3 34B4 errorß for each
3 However, in the present context, adjustments for additional effects such as the dose
levels are needed; thus we create additional covariate vectors D"ß ÞÞÞß D7 and fit a set of linearmodels of the form B
4Á3 34B4 errorÞ PLS regression is used to estimate
the coefficients +3"ß ÞÞÞß +37ß ,3"ß ÞÞÞß ,3ß3"ß ,3ß3"ß ÞÞÞß ,3: based on the design matrix formed bythe covariates in the PLS model. The PLS scores are computed based on the estimates,3"ß ÞÞÞß ,3ß3"ß ,3ß3",ÞÞÞß ,3:. The details of the method for computing the PLS regressionestimates of the regression coefficients and their conversion to PLS scores are omitted in thisextended abstract; these were along the same lines as Pihur et al. (2008). A symmetrizedestimate of regression coefficient , is taken as the PLS association score =35 œ Ð ,
Once the connectivity scores are computed for each network, a permutation test is
performed to test for differential connectivity of the class of all genes or the test for a single
denotes the connectivity scores between genes
3 and 5 for networks 1
and 2, respectively. The test statistic for the class of all genes Y with cardinality 0 is
HÐ=Ð"Ñß =Ð#ÑÑ
and the test statistic for a single gene 1 is
Ð=Ð"Ñß =Ð#ÑÑß
where H computes the distance between the connectivity scores. We have worked with theP
" -distance HÐ=
ß = Ñ œ l= = rather than the more commonly used P# -distance
leading to a more robust analysis. The permutation test is performed by randomly assigningthe labels to each observation in the data set formed by combining the observations from bothnetworks.
4. Results For each of the 131 drugs, tests for differential connectivity of the networks on the
set of all 473 non-differentially expressed genes (1) were performed using 1000 permutations
based on the P" distance function and the PLS connectivity scores. No significant differences
in the overall connectivity scores of the networks of this set of 473 genes were found for 77 of
the 131 drugs at a 5% significance level. These drugs are listed in Table 1.
acarbose disopyramide nimesulide
acetamidofluorene disulfiram nitrosodiethylamine acetaminophen doxorubicin papaverine acetazolamide enalapril penicillamine adapin erythromycin ethylsuccinate phenacetin amitriptyline ethambutol phenobarbital bendazac ethinylestradiol phenylanthranilic acid benziodarone ethionamide propylthiouracil bromoethylamine etoposide puromycin aminonucleoside bucetin famotidine quinidine captopril fenofibrate simvastatin carboplatin fluphenazine sulindac cephalothin flutamide sulpiride chloramphenicol gentamicin tamoxifen chlormadinone griseofulvin tannic acid chlormezanone hydroxyzine terbinafine chlorpheniramine imipramine tetracycline chlorpromazine labetalol theophylline chlorpropamide lomustine thioridazine ciprofloxacin lornoxicam ticlopidine clomipramine mefenamic acid tiopronin colchicine meloxicam tolbutamide cyclosporine A metformin triamterene danazol methyltestosterone triazolam dantrolene mexiletine trimethadione
diltiazem nifedipine
Table 1: Drugs with similar connectivity scores in the two networks.
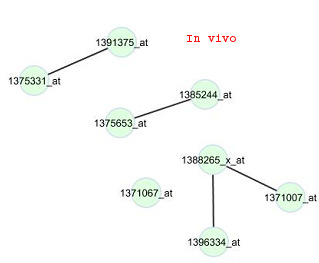
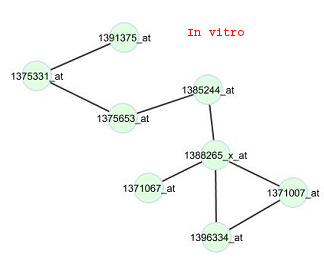
Even among the 54 drugs for which the set of all genes are significantly different in
terms of overall network connectivities, there are many genes that are not significantlydifferent in terms of individual connectivity scores in the two networks at a 5% level. Tests forthe significance difference of the connectivity score of each individual gene within the network(2) were performed for the 54 drugs, and there were 35 genes that were not differentiallyconnected for at least 70% of the drugs. These genes are shown in Table 2.
GENE prop. GENE prop. GENE prop. GENE prop. GENE prop.
1385656_at 0.833 1397371_at 0.759 1395446_at 0.741 1381550_at 0.722 1392859_at 0.7041395874_at 0.815 1396604_at 0.759 1375063_at 0.741 1370626_at 0.722 1388033_at 0.7041378788_at 0.796 1392389_at 0.759 1396731_at 0.722 1398741_at 0.704 1385031_at 0.7041396340_at 0.778 1391493_at 0.759 1385655_at 0.722 1398675_at 0.704 1383272_at 0.7041393711_at 0.778 1368887_at 0.759 1385589_at 0.722 1397850_at 0.704 1383195_at 0.7041391313_at 0.778 1368854_at 0.759 1384683_at 0.722 1397720_at 0.704 1381502_at 0.7041398707_at 0.759 1397339_at 0.741 1384061_at 0.722 1395490_at 0.704 1377391_at 0.704
Table 2: Genes not differentially expressed for at least 70% of the remaining 54 drugs. The respective
gene names (probe set IDs) and proportion of drugs with similar connectivity scores for that gene in the
in vivo and in vitro networks.
In order to characterize the 473 genes which have shown no significant difference
between the in viv
o and in vitr
o types with more than 80% of the drugs we used functional
annotation tool DAVID (Huang et al., 2009a; 2009b). Results of that analysis for the top fivefunctional clusters out of the 473 genes are given in Table 3. Most of the genes in the firstfunctional cluster are involved in neuron development, neuron differentiation, neuronprojection morphogenesis and cell morphogenesis activities. The genes in the second mostimportant cluster are involved with proteins in cell-cell junctions of multi-cellular species andalso most of them are associated with some synaptic activities. The third most importantfunctional cluster of the genes are associated with epidermal growth factor (EGF) proteins.
Figure 1: In viv
o and in vitr
o networks for
cluster 4 and the drug phenylbutazone. Edges
are displayed for gene pairs with connectivity
scores (rescaled so that the largest score for the
network is 1 in magnitude) greater than 0.5 in
Table 3: Tests of differential connectivity for
the top 5 clusters obtained from the DAVID
Functional Annotation Tool. The last columnshows the percentages of drugs for which the
corresponding sub-networks were not
Next, we reconstructed the networks separately for each functional cluster. These networkshad fewer significant differences between the in viv
o and in vitr
o types than the overall
networks. As seen in the Table 3, the difference between the in viv
o and in vitr
o networks are
not statistically significant for at least 87% of the drugs among these top five clusters.
We also annotated 35 genes for each of which the individual network connectivity
score between the in viv
o and in vitr
o types remained unchanged in spite of having
significantly different total gene set network connectivity scores under the treatment of 54drugs. With DAVID annotation tool we figured that all these 35 genes are in one functionalcluster and they are associated with cellular macromolecular complex assembly.
Lastly, we wanted to illustrate how these sub-networks behave for a given drug.
Figure 1 illustrates the constructed in vivo and in vitr
o networks for the genes in cluster 4 for
phenylbutazone, a non-steroidal anti-inflammatory drug (NSAID). For these networks, thetest for differential connectivity is not significant (p-value is 0.42). All edges in the in vivonetwork also appear in the in vitr
o network, and only 4 edges in the in vitr
o network do not
appear in the in viv
o network.
5. Conclusion A comprehensive view of the in viv
o - in vitr
o bridge of the genes using the
rat microarray TGP study under all the drugs is undertaken. We not only provide the similarityof individual gene expression pattern but also that of the association networks under in vivoand in vitro experiments. The systems are scrutinized in terms of overall network connectivityand also in terms of individual gene connectivity. We use PLS based association scoresadjusted for sacrifice time and dosage followed by a permutation based statistical test withthose scores. Since we are trying to identify genes that are not different, a conservativeapproach in this context will be not to apply a multiple testing p-value correction unlike typicalgene expression studies where the goal is to identify genes that are differentially expressedand/or connected under two biological conditions. It is interesting to observe that, similar toUehara et al. (2010) who studied three of the drugs, none of the bridging genes that we foundare involved with cell proliferation and apoptosis.
A potential limitation of our study is that our findings are based on a specific type of
statistical model. In the future we plan to undertake additional investigation where networksare constructed by fitting other types of predictive models such as lasso (Tibshirani, 1996) andelastic net (Zou and Hastie, 2005) and the results are compared.
The findings must be interpreted carefully. First of all, we have highlighted the genes
which were not significantly different. However it does not quite imply that in viv
o and in
vitro studies are completely interchangeable since there are genes that show differentialexpression and network profiles in the two networks. Furthermore, lack of statisticalsignificance does not necessarily imply that the objects under comparison are indeed equal.
References
Gill, R., Datta, S., and Datta, S. (2010). BMC Bioinformatics, 11, 95.
Gill, R., Datta, S., and Datta, S. (2012). http://CRAN.R-project.org/package=dna
Pihur, V., Datta, S., and Datta, S. (2008). Bioinformatics, 24, 561-568.
Uehara T., Ono A., Maruyama T., Kato I., Yamada H., Ohno Y., Urushidani T. (2010). Mol.
Nutr. Food Res.,
54 218-227.
Huang, D.W., Sherman, B. T., Lempicki, R. A. (2009a). Nature Protoc., 4, 44-57.
Huang, D. W., Sherman, B. T., Lempicki, R. A. (2009b). Nucleic Acids Res., 37, 1-13.
Zou, H., Hastie, T. (2005). J. Royal. Statist. Soc. B.,
67 301–320.
Tibshirani, R. (1996). J. Royal. Statist. Soc. B., 58, 267-288.
Source: http://dokuwiki.bioinf.jku.at/lib/exe/fetch.php/gill.pdf
MONOGRAPHIE DE PRODUIT PrTRINTELLIXMC Vortioxétine (sous forme de bromhydrate de vortioxétine) Comprimés dosés à 5, 10 et 20 mg Lundbeck Canada Inc. Date de rédaction : 1000, rue De La Gauchetière Ouest, Montréal (Québec) Numéro de contrôle de la présentation : 159019 Monographie de TRINTELLIX Page 1 de 46
e-mail: [email protected] J Babol Univ Med Sci; 11(3); Aug-Sep 2009 The Effect of Sucralfate in Prevention ; N. Ahmadloo, et al RTOG/ EORTC Kneebone, Martenson J Babol Univ Med Sci; 11(3); Aug-Sep 2009 The Effect of Sucralfate in Prevention ; N. Ahmadloo, et al The Effect of Sucralfate in Prevention of Radiation- Induced Acute Proctitis